Hybrid Legal Document Review: Where Human and Artificial Intelligence Meet
AI is in many ways still in its infancy, and it's important to realize that platforms utilizing this technology are heavily dependent on constant human interaction and training.
February 27, 2020 at 03:10 PM
12 minute read
This article appeared in Cybersecurity Law & Strategy, an ALM publication for privacy and security professionals, Chief Information Security Officers, Chief Information Officers, Chief Technology Officers, Corporate Counsel, Internet and Tech Practitioners, In-House Counsel. Visit the website to learn more.
We hear a lot about the potential of artificial intelligence and machine learning to make the e-discovery process faster and more accurate — and in many ways, the technology is living up to these promises. But the human element remains a critical component, essentially creating a hybrid legal document review process that blends human and artificial intelligence to achieve the optimal results.
Our industry has been revolutionized by AI's ability to quickly dig through large amounts of data, locate relevant information and make review calls with a high degree of precision. Without this technology's ability to organize files and data and present them in a way that allows us to identify similarities, trends and outliers, we would simply be unable to cost-effectively manage the sheer volume of data that we encounter during the e-discovery process.
Yet, AI is in many ways still in its infancy, and it's important to realize that platforms utilizing this technology are heavily dependent on constant human interaction and training. As AI and machine learning technology continue to develop, there will come a time when computers will be able to perform increasingly larger portions of projects with little or no human input, delivering a higher level of quality than humans can achieve on their own.
But for the foreseeable future, the human role in e-discovery will be a vital part of the process, and a "hybrid" or combined technology-plus-people approach to e-discovery will continue to be the industry's most effective model.
Let's take a closer look at how human effort and artificial intelligence work together, and the ways in which each relies upon the other.
|AI and Machine Learning
Before going any further, let's first define what we're referring to when we talk about "artificial intelligence." It's a bit of a misnomer to use that term, since current e-discovery platforms don't have self-awareness or independent intelligence and are useless without the guidance of humans. Machine learning, a subset of AI that has the ability to make intelligent predictions about data after undergoing initial human-driven training, is really what's being utilized in e-discovery today. Depending on how well it's trained, a predictive machine-learning model can deliver more accurate results than a human in a much shorter amount of time.
In e-discovery, we use machine learning technology to wade through enormous data sets in search of relevant documents for legal matters. This process, called Technology Assisted Review (TAR), starts with a human reviewing a certain number of documents and coding them as either relevant or non-relevant. Building from that "seed set," the TAR software begins to categorize other documents in a similar way, becoming more accurate with the help of additional human input. Of course, it can become more complicated, with many additional facets and issues being decided as well, but the same basic concept still lies at the heart of the process.
|Garbage In, Garbage Out
Typically, most competent document review services offered by e-discovery providers today will include at least some TAR element as part of the managed review process. When looking at those solutions, it is critical to ensure that the correct combination of elements — technology, people and process — are aligned in any such service to ensure the success of the overall review effort. And even with the newest technology, it's still the people involved on which it all hinges.
Though newer iterations of TAR software include modern features and approaches to help address some of the early shortcomings — such as using a process called continuous active learning to eliminate the reliance on a single initial seed set, as referenced above — even those newer systems' effectiveness still ultimately hinge on the quality of the user input. If the user makes errors in coding documents, or doesn't code enough documents, or doesn't review enough variety of document types to train the software sufficiently, it's not going to be as useful as it could be — or worse.
It is the latest example of one of the oldest adages in technology: Computer systems tend to amplify the quality or defectiveness of the input (or "training," in TAR-speak). So, if the initial work is "garbage," that's exactly what the output will be too, only amplified. Or more simply stated: garbage in, garbage out.
|Power of the People
While a document reviewer's subject matter expertise is an essential element of any TAR-enabled review process, so are the reviewer's technical experience and skill set, which matter just as much, if not more. That's why it's important for legal document reviewers to master not just document review best practices and various subject matters, but also to become experts in a variety of different TAR and legal analytics technologies. Even if they've never studied anything even close to statistics and algorithms in law school, by embracing these new technologies they can arguably understand their impact and optimal use better than some of the programmers behind the algorithms themselves, and that makes all the difference in the world.
At my company, that essential, hybrid blend of machine learning systems with the right technical and subject matter experts has shown us time and time again to be the key to a truly successful TAR review project. And the combination doesn't just reduce costs, it also further bolsters accuracy, increases production speed, helps more quickly evaluate case issues and reduces the entire case timeline, making the overall legal dispute process much more efficient in all aspects.
It's truly that human element that makes the entire TAR process work to its best potential. Also, Courts are more likely to embrace the use of TAR technologies in a case when shown that there is a highly skilled and experienced team backing up the AI technology.
|But the Old Ways Aren't Dead
To be clear, TAR technology doesn't necessarily mean the end of the traditional search-term approach. Search terms still can be extremely valuable, especially in the early stages of culling and key document identification. We often incorporate various types of keyword searches to locate relevant documents in a large data set. Among them are Boolean searches, which involve modifier words like AND, OR and NOT, and proximity searches, which examine how close certain search terms are to each other.
The limitation of such "static" searches is that they scan words simply as a series of letters without analyzing their larger context. Static searches can still be useful at times, especially with well-focused search terms and phrases that can help quickly pinpoint specific documents, discussion, or the like. Then, the TAR technologies can help expand on what's found in a larger context. When properly used, the two approaches can and do work together to generate results that exceed anything either could do alone. That's essentially what made Google what it is today. It's still based on searches, but it's the algorithms that took those simple searches to a whole new level.
|AI Is Getting Emotional Now Too
Another emerging branch of AI worth noting, called emotionally intelligent AI, takes the technology a step further by searching for language that can indicate certain emotional states, among them positivity and negativity, opportunity, intent, rationalization and pressure. It factors in the presence of specific words, the way phrasing and punctuation are used, and even contextual information like the time of day to discern the emotions being conveyed in a particular document.
In contrast with other forms of AI, there's no training period required for that technology to work, as it is not dependent on the subject matter of a given case, so it can deliver results from the very beginning of the e-discovery process. We expect this technology to become a common fixture in e-discovery platforms, and additional experimentation with its capabilities will help it further show its value.
|There Are Still Shortcomings
Even with the many advancements in AI-based technology used in e-discovery software and legal technology, there are still areas that need attention. For instance, tables and graphics are not easily scannable by automated systems. Excel spreadsheets, in particular, can be particularly problematic if they're complex or contain a large amount of text. In cases like these, the human side of the equation — specifically, relying on experienced attorney reviewers — becomes more important than ever.
Also, more advanced recognition algorithms, such as identifying images or skin tone in graphics documents and objects in multimedia evidence, are still lacking. Though the technologies exist, there is still no integration into current legal document review platforms, and even when utilized, false positives are common. Currently, people are still an essential element of ensuring that those graphical and multimedia file types are reviewed properly.
|The Human Element Is Still Key
In the end, without the human element, TAR, machine learning and text analytics are merely computer software programs, created by fallible humans, attempting to make the best decisions the algorithms allow.
And because the results of those processes are used in legal proceedings to make decisions that have a major impact, not just on legal issues generally, but on the futures of people, governments and corporations, courts are likely to continue to require that the human element — experienced legal document reviewers and technology experts — continue to act as a quality control gateway for the technology employed by the algorithms.
Humans also bring a number of special qualities to the document review process — among them, institutional knowledge, experience in specific legal areas and natural problem-solving skills — none of which can yet be replicated at the same level of quality by an algorithm. They also bring backgrounds in areas such as intellectual property, antitrust, second requests, product liability, general commercial litigation, contract disputes, mass torts, governmental investigations and class actions — all of which are difficult or impossible for a computer algorithm to develop or replicate in a vacuum.
As we said before, these systems aren't truly artificial intelligence, at least not in the science-fiction sense. They cannot truly think on their own. So, human intelligence still rules the ultimate result.
|TAR and AI Amplify the Importance of Expertise.
Although some e-discovery companies use review teams composed entirely of temporary workers, we've found it much more effective to utilize a smaller full-time team of technology trained, bar-admitted attorneys to power TAR reviews. The expertise of even a small, highly skilled team will increase the power of the human element on which all TAR reviews are built and obtain results that much larger teams couldn't ever touch.
A full-time, expert and dedicated core document review team can also raise the overall level of quality and consistency, reduce the time needed to ramp up, and reduce concerns over not just inexperienced mistakes, but confidentiality and security as well. Simply put, you end up with a much more useful result from a smaller, more manageable group, and for a lot less cost.
Yet, that shouldn't be a surprise. After all, isn't that the ultimate promise of automation and technology: to provide a better, more efficient product with less manual effort? That's exactly what happens when you do TAR right — and with the right team.
And for companies that face routine litigation, that can be amplified even further. A consistent review team's institutional knowledge can be leveraged even more so to address unfamiliar or unexpected situations head-on, helping to increase the quality of the data fed into the TAR algorithms, and thus, the results. If they code a particular client's documents on a recurring basis, they'll also be familiar with the company's product lines, nicknames and code words, for example. In addition, they'll have an existing working relationship with general counsel, outside counsel and paralegals, making it easier to untangle specific complex issues and questions that might come up. All that experience and knowledge is leveraged by the TAR technologies to produce a better end result.
By harnessing the power of AI and machine learning, and integrating it into a human-managed process from beginning to end — legal hold through data collection, processing, reviewing and production — e-discovery can reach a high level of efficiency and quality while eliminating added costs, such as idle reviewer time and excess staffing.
But until the day comes when AI is able to truly think in the way that humans do — learning facts, methods and processes, and then applying this newfound knowledge to projects going forward — hybrid review will continue to be the ideal combination of the unique qualities that humans and technology both bring to the table.
*****
Brian Schrader, Esq., is President & CEO of BIA (www.biaprotect.com), a leader in reliable, innovative and cost-effective ediscovery services. With early career experience in information management, computer technology and the law, Brian co-founded BIA in 2002 and has since developed the firm's reputation as an industry pioneer and a trusted partner for corporations and law firms around the world. He can be reached at [email protected].
This content has been archived. It is available through our partners, LexisNexis® and Bloomberg Law.
To view this content, please continue to their sites.
Not a Lexis Subscriber?
Subscribe Now
Not a Bloomberg Law Subscriber?
Subscribe Now
NOT FOR REPRINT
© 2024 ALM Global, LLC, All Rights Reserved. Request academic re-use from www.copyright.com. All other uses, submit a request to [email protected]. For more information visit Asset & Logo Licensing.
You Might Like
View All
Will Trump Be a Boost to Quinn Emanuel's Fortunes in China?

Pa. Judicial Nominee Advances While Trump Demands GOP Unity Against Biden Picks
4 minute read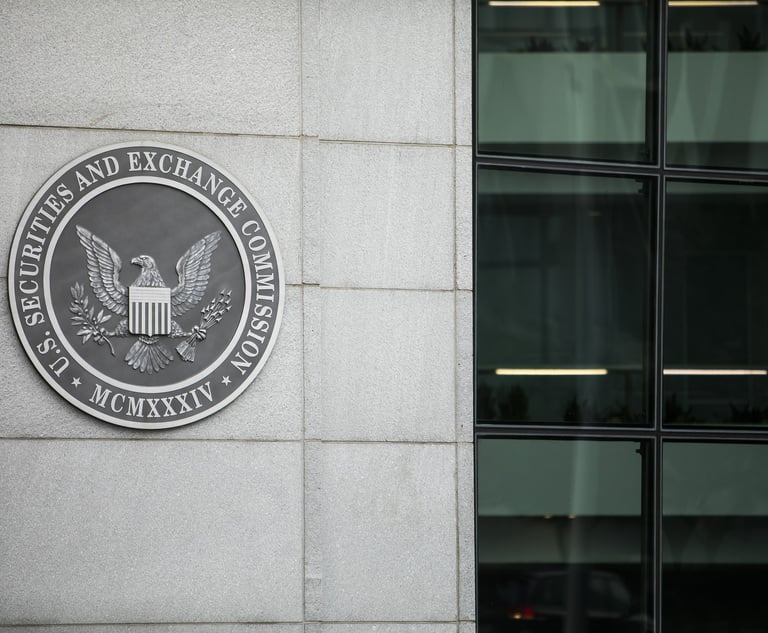
Trump's SEC Overhaul: What It Means for Big Law Capital Markets, Crypto Work
Trending Stories
Who Got The Work
Michael G. Bongiorno, Andrew Scott Dulberg and Elizabeth E. Driscoll from Wilmer Cutler Pickering Hale and Dorr have stepped in to represent Symbotic Inc., an A.I.-enabled technology platform that focuses on increasing supply chain efficiency, and other defendants in a pending shareholder derivative lawsuit. The case, filed Oct. 2 in Massachusetts District Court by the Brown Law Firm on behalf of Stephen Austen, accuses certain officers and directors of misleading investors in regard to Symbotic's potential for margin growth by failing to disclose that the company was not equipped to timely deploy its systems or manage expenses through project delays. The case, assigned to U.S. District Judge Nathaniel M. Gorton, is 1:24-cv-12522, Austen v. Cohen et al.
Who Got The Work
Edmund Polubinski and Marie Killmond of Davis Polk & Wardwell have entered appearances for data platform software development company MongoDB and other defendants in a pending shareholder derivative lawsuit. The action, filed Oct. 7 in New York Southern District Court by the Brown Law Firm, accuses the company's directors and/or officers of falsely expressing confidence in the company’s restructuring of its sales incentive plan and downplaying the severity of decreases in its upfront commitments. The case is 1:24-cv-07594, Roy v. Ittycheria et al.
Who Got The Work
Amy O. Bruchs and Kurt F. Ellison of Michael Best & Friedrich have entered appearances for Epic Systems Corp. in a pending employment discrimination lawsuit. The suit was filed Sept. 7 in Wisconsin Western District Court by Levine Eisberner LLC and Siri & Glimstad on behalf of a project manager who claims that he was wrongfully terminated after applying for a religious exemption to the defendant's COVID-19 vaccine mandate. The case, assigned to U.S. Magistrate Judge Anita Marie Boor, is 3:24-cv-00630, Secker, Nathan v. Epic Systems Corporation.
Who Got The Work
David X. Sullivan, Thomas J. Finn and Gregory A. Hall from McCarter & English have entered appearances for Sunrun Installation Services in a pending civil rights lawsuit. The complaint was filed Sept. 4 in Connecticut District Court by attorney Robert M. Berke on behalf of former employee George Edward Steins, who was arrested and charged with employing an unregistered home improvement salesperson. The complaint alleges that had Sunrun informed the Connecticut Department of Consumer Protection that the plaintiff's employment had ended in 2017 and that he no longer held Sunrun's home improvement contractor license, he would not have been hit with charges, which were dismissed in May 2024. The case, assigned to U.S. District Judge Jeffrey A. Meyer, is 3:24-cv-01423, Steins v. Sunrun, Inc. et al.
Who Got The Work
Greenberg Traurig shareholder Joshua L. Raskin has entered an appearance for boohoo.com UK Ltd. in a pending patent infringement lawsuit. The suit, filed Sept. 3 in Texas Eastern District Court by Rozier Hardt McDonough on behalf of Alto Dynamics, asserts five patents related to an online shopping platform. The case, assigned to U.S. District Judge Rodney Gilstrap, is 2:24-cv-00719, Alto Dynamics, LLC v. boohoo.com UK Limited.
Featured Firms
Law Offices of Gary Martin Hays & Associates, P.C.
(470) 294-1674
Law Offices of Mark E. Salomone
(857) 444-6468
Smith & Hassler
(713) 739-1250