Artificial Intelligence: The Fastest Moving Technology
If artificial intelligence is truly our fasting moving technology, the law has been lagging far behind. Addressing the emerging legal issues requires an understanding of the technology and how it works. In his Technology Law column, Peter Brown examines how AI functions and some of its legal implications.
March 09, 2020 at 12:30 PM
9 minute read
"In our business, we talk about emerging technologies and how they impact society. We've never seen a technology move as fast as AI has to impact society and technology. This is by far the fastest moving technology that we've ever tracked in terms of its impact and we're just getting started."
Paul Daugherty, CTO, Accenture
If artificial intelligence (AI) is truly our fasting moving technology, the law has been lagging far behind. Addressing the emerging legal issues requires an understanding of the technology and how it works. In my prior article ("Waking Up to Artificial Intelligence," NYLJ (Feb. 11, 2020)), I discussed the concepts of machine learning and deep learning. This column will continue to examine how AI functions and some of its legal implications.
Natural Language Processing
Natural Language Processing (NLP) refers to the ability of a computer system to understand human speech in a language such as English, in contrast to understanding an artificial computer language that is used to create a computer program. NLP enables a computer system to process speech in context with a useful understanding of language features such as grammar, structure, context and meaning. On a deeper level, NLP enables a computer system to interact with humans in a more "natural" way, by receiving human language input (such as directly receiving human speech), and returning output in the form of human language. NLP is increasingly used in many contexts from smart speakers/home assistants like the Amazon Echo, Google Assistant or Siri, to automated telephone call centers.
For an NLP system to work it must initially break down the spoken word into text which can be processed and interpreted. These voice-based systems generally use a statistical system known as Hidden Markov Models (HMM). The HMM system uses mathematical models to interpret the spoken word and translate that information into text. In practice the HMM systems will review 10-20 millisecond clips of speech and look for phonemes (the smallest unit of speech) to compare with its pre-recorded files.
However, to understand speech the system must interpret the words and their context. The typical system will break down each word and determine whether it is a verb, adjustive, noun etc. The words are then processed through algorithms that incorporate series of coded grammar rules that interpret the context of the speech. The end result is the system's ability to classify the spoken words for use in the application. The NLP system may then search for an appropriate response to a question or identify products on a website that it is marketing in response to a customer inquiry.
Finally, the system engages in semantic analysis to interpret a logic behind a spoken sentence. To accomplish this, the HMM system breaks down each sentence into its basic structure and the semantic analysis provides the context of the speech. The semantic analysis is a key process in the use of speech in AI. The combination of HMM and semantic analysis provides a powerful interface with an AI system and makes it both accessible and highly functional. Since these systems improve with exposure to large volumes of information, i.e., speech samples, the NLP systems will only become more useful and nuanced over time.
Image and Facial Recognition
A human can easily recognize and distinguish the content of digital images, but image recognition is a difficult task for a computer, when one is trying to program for specific features. Image recognition is a critical feature for the practical deployment of many AI systems, like vehicles moving in a warehouse or on the road.
For example, self-driving automobile technology must learn to "understand" its visual environment in order to successfully navigate such varied situations as city streets and country roads. Similarly, personal care robots must learn to distinguish between individual humans and recognize the various body parts of humans, and navigate in ordinary human space. To achieve widespread practical utility, AI systems must be able to process and understand both the text and images within documents the AI system is instructed to review.
Advances in image recognition have benefitted from the significant growth in computational power available to researchers, the collections of massive image databases with object labels (aka training data sets), and advances in general machine learning tools such as deep learning and neural networks. In 2015, a team from Microsoft, using "deep neural network" technology, achieved a significant milestone when its image recognition technology performed an image classification challenge with an error rate of only 3.6%, as compared to a human error rate of 5.1%
Facial recognition technology, a subset of image recognition, is already in active use. To the great annoyance of some individuals, social media sites scan photos uploaded by users to detect human faces, and often identify them. Current systems can identify an individual in a crowd or against an intricate background. To accomplish this task the facial recognition system breaks down the geometry of a face into various landmarks or nodal points. A human face can be measured using up to 80 nodal points. These include: the distance between eyes; the shape of the check; the contours of the jaw line; and, the width of the nose. Each of the landmarks are measured. The result is numerical code, which becomes a facial signature in the system's database.
When the system is instructed to identify a facial signature, it is compared against the mathematical signatures in the system's database. It must be noted that over 100 million Americans have their images in one or more police databases. Of equal significance is the voluntary postings of personal photographs of hundreds of millions of individuals. Various commercial and government entities scrape these images to be included in their databases. For example, Facebook has a feature that scans a group photograph and it will identify by name the family members or friends in the posted photograph.
Facial recognition occurs when the system compares the known facial signature to the massive institutional databases of facial images. Systems deploying facial recognition software are already used in many contexts, including the identification of individuals in airports by the Department of Homeland Security; replacing office security badges with facial recognition; assisting local police in identifying criminal suspects; and surveillance in retail stores seeking to deter shoplifters.
AI and Personal Privacy
Privacy risks are a recurring theme in discussions of new and improving digital technologies and AI is no exception. Over the past several decades, digitization of government records, digital commerce, mobile communications and social media, have all generated enormous databases of information about individuals, creating well-recognized risks to personal privacy. With the ability of AI systems to mine those databases and generate new insights into individual interests, behaviors and interactions, the risks to personal privacy have grown exponentially.
In 2010, the U.S. Court of Appeals for the District of Columbia Circuit issued an opinion that acknowledges the privacy risks involved in accumulating a database of information about an individual, even where each item in the database, if considered separately, is a matter of public knowledge. United States v. Maynard, 615 F.3d 544 (D.C. Cir. 2010). In Maynard, the appeals court concluded that short-term GPS tracking of a drug trafficking suspect might not require a warrant, but such tracking sustained over a month required a warrant. The court reasoned that the warrant requirement was triggered because the accumulation of information about the suspect's movements over time revealed more than any of the individual pieces of data that were collected.
Although the U.S. Supreme Court affirmed the ruling in Maynard on different grounds, the justices acknowledged the risks to personal privacy involved in the digital capture and accumulation of data. Justice Sotomayor's concurring opinion suggested that the principle that an individual has no expectation of privacy in information voluntarily disclosed might need to be reconsidered:
This approach is ill suited to the digital age, in which people reveal a great deal of information about themselves to third parties in the course of carrying out mundane tasks. People disclose the phone numbers that they dial or text to their cellular providers; the URLs that they visit and the e-mail addresses with which they correspond to their Internet service providers; and the books, groceries, and medications they purchase to online retailers. U.S. v. Jones, 132 S. Ct. 945, 957 (Sotomayor, J., concurring).
Justice Sotomayor's concurring opinion, like the lower court ruling, implicitly recognize that the accumulation of information about citizens is a serious risk to privacy.
The ability of AI systems to mine databases containing personal information and facial images raises these risks exponentially, because AI technology can extract revealing insights that are not otherwise available through existing technologies. When considered in the context of improving medical diagnoses, this capacity of AI is a good thing: Processing masses of information about medical outcomes of cancer patients may yield a life-saving diagnosis for a single patient. But the same data-crunching capacity of AI systems could potentially be used to the detriment of an individual, e.g., by an insurance company in making biased decisions about coverage, or by an employer to deny employment. The risks remain even if the data is stored in an "anonymous" fashion. AI technology has been shown to be adept at re-identifying individuals, in data that had otherwise been anonymized. In the near future, the useful advances in AI technology may need to be regulated to protect against the threats to personal privacy.
Peter Brown is the principal at Peter Brown & Associates. He is a co-author of "Computer Law: Drafting and Negotiating Forms and Agreements" (Law Journal Press). Jonathan Kaplan of Kaplan IP Law PLLC in Camas, Washington, contributed to the preparation of this article.
This content has been archived. It is available through our partners, LexisNexis® and Bloomberg Law.
To view this content, please continue to their sites.
Not a Lexis Subscriber?
Subscribe Now
Not a Bloomberg Law Subscriber?
Subscribe Now
NOT FOR REPRINT
© 2025 ALM Global, LLC, All Rights Reserved. Request academic re-use from www.copyright.com. All other uses, submit a request to [email protected]. For more information visit Asset & Logo Licensing.
You Might Like
View All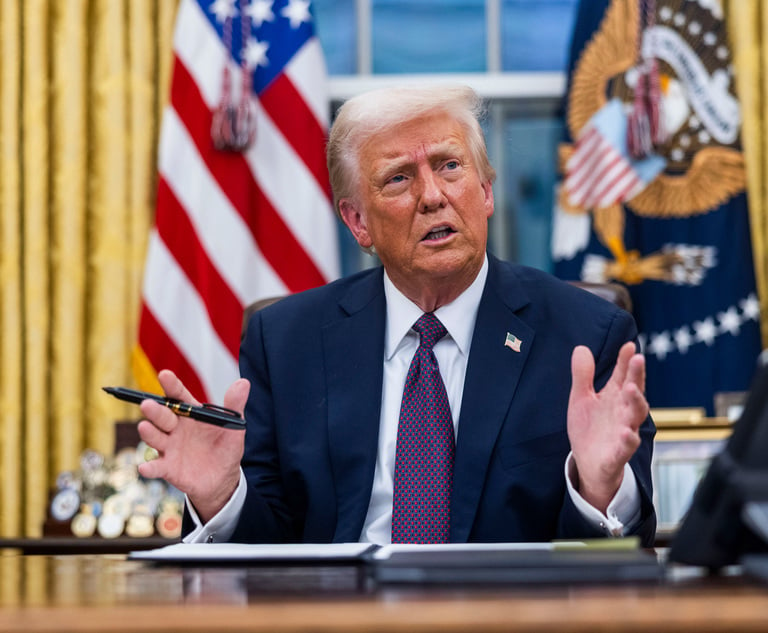
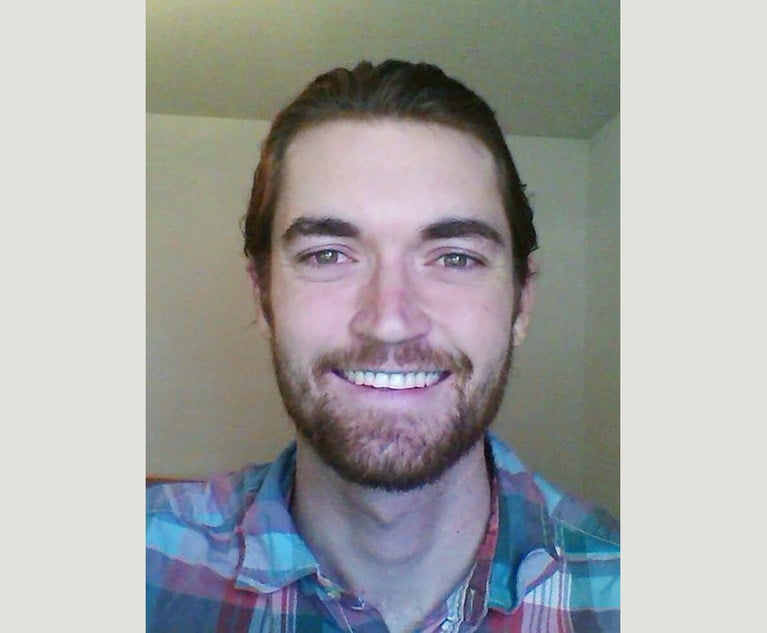
Silk Road Founder Ross Ulbricht Has New York Sentence Pardoned by Trump
3 minute read
Walt Disney, IBM Denied High Court Review of Old NY Franchise Tax Law
3 minute read
‘Issue of First Impression’: New York Judge Clears Coinbase Appeal Amid Crypto Regulatory Clash
4 minute readLaw Firms Mentioned
Trending Stories
- 1Meet the New President of NY's Association of Trial Court Jurists
- 2Lawyers' Phones Are Ringing: What Should Employers Do If ICE Raids Their Business?
- 3Freshfields Hires Ex-SEC Corporate Finance Director in Silicon Valley
- 4Meet the SEC's New Interim General Counsel
- 5Will Madrid Become the Next Arbitration Hub?
Who Got The Work
J. Brugh Lower of Gibbons has entered an appearance for industrial equipment supplier Devco Corporation in a pending trademark infringement lawsuit. The suit, accusing the defendant of selling knock-off Graco products, was filed Dec. 18 in New Jersey District Court by Rivkin Radler on behalf of Graco Inc. and Graco Minnesota. The case, assigned to U.S. District Judge Zahid N. Quraishi, is 3:24-cv-11294, Graco Inc. et al v. Devco Corporation.
Who Got The Work
Rebecca Maller-Stein and Kent A. Yalowitz of Arnold & Porter Kaye Scholer have entered their appearances for Hanaco Venture Capital and its executives, Lior Prosor and David Frankel, in a pending securities lawsuit. The action, filed on Dec. 24 in New York Southern District Court by Zell, Aron & Co. on behalf of Goldeneye Advisors, accuses the defendants of negligently and fraudulently managing the plaintiff's $1 million investment. The case, assigned to U.S. District Judge Vernon S. Broderick, is 1:24-cv-09918, Goldeneye Advisors, LLC v. Hanaco Venture Capital, Ltd. et al.
Who Got The Work
Attorneys from A&O Shearman has stepped in as defense counsel for Toronto-Dominion Bank and other defendants in a pending securities class action. The suit, filed Dec. 11 in New York Southern District Court by Bleichmar Fonti & Auld, accuses the defendants of concealing the bank's 'pervasive' deficiencies in regards to its compliance with the Bank Secrecy Act and the quality of its anti-money laundering controls. The case, assigned to U.S. District Judge Arun Subramanian, is 1:24-cv-09445, Gonzalez v. The Toronto-Dominion Bank et al.
Who Got The Work
Crown Castle International, a Pennsylvania company providing shared communications infrastructure, has turned to Luke D. Wolf of Gordon Rees Scully Mansukhani to fend off a pending breach-of-contract lawsuit. The court action, filed Nov. 25 in Michigan Eastern District Court by Hooper Hathaway PC on behalf of The Town Residences LLC, accuses Crown Castle of failing to transfer approximately $30,000 in utility payments from T-Mobile in breach of a roof-top lease and assignment agreement. The case, assigned to U.S. District Judge Susan K. Declercq, is 2:24-cv-13131, The Town Residences LLC v. T-Mobile US, Inc. et al.
Who Got The Work
Wilfred P. Coronato and Daniel M. Schwartz of McCarter & English have stepped in as defense counsel to Electrolux Home Products Inc. in a pending product liability lawsuit. The court action, filed Nov. 26 in New York Eastern District Court by Poulos Lopiccolo PC and Nagel Rice LLP on behalf of David Stern, alleges that the defendant's refrigerators’ drawers and shelving repeatedly break and fall apart within months after purchase. The case, assigned to U.S. District Judge Joan M. Azrack, is 2:24-cv-08204, Stern v. Electrolux Home Products, Inc.
Featured Firms
Law Offices of Gary Martin Hays & Associates, P.C.
(470) 294-1674
Law Offices of Mark E. Salomone
(857) 444-6468
Smith & Hassler
(713) 739-1250