
When Machines Do the Hiring, Some See a Need to Revamp Job Bias Law
Increased use of algorithms, or machine learning, to evaluate job seekers is expected to result in more unintended discrimination in hiring.
June 12, 2019 at 06:07 PM
7 minute read
As corporations increase their reliance on automated programs to evaluate potential job candidates, lawyers can expect to hear more about an unintended byproduct: algorithmic discrimination.
Increased use of algorithms, or machine learning, to evaluate job seekers is expected to result in more unintended discrimination in hiring. But there's a debate among legal scholars about whether job applicants denied employment based on protected-class discrimination by a resume-reading artificial intelligence can find a remedy under existing laws.
The appeal of software that evaluates candidates is obvious for any corporation that gets thousands of resumes for a single opening. And the companies selling artificial intelligence tools as a way to ease the drudgery of hiring promise their products can reduce interpersonal bias that can result from face-to-face contact with a human resources manager. Yet those same artificial intelligence applications are not immune from perpetuating existing biases.
As Stephanie Bornstein of the University of Florida Levin College of Law wrote in a research paper, “If the underlying data on which an algorithm relies is itself biased, incomplete, or discriminatory, the decisions it makes have the potential to reproduce inequality on a massive scale.”
Amazon scrapped an algorithm it developed to evaluate job applicants after discovering it showed a preference for male candidates. The algorithm made decisions based on its evaluation of data from Amazon's current, male-dominated workforce, and taught itself that male candidates were preferable and gave less credit to female applicants.
So far, the plight of job seekers turned away because of bias from an algorithm is mostly hypothetical. But the fate of job applicants, real or theoretical, spurned by machine-learned bias has drawn wide interest in the legal field.
Courts currently divide employment discrimination into two categories—disparate treatment and disparate impact. But some lawyers believe the established way of looking at discrimination appears may need a redo.
Neither the disparate treatment nor disparate impact theories seem to bar discrimination from a machine, said Charles Sullivan, who teaches a course on employment discrimination at Seton Hall University School of Law in Newark.
An algorithm that excludes women as seen by Amazon, or the employer that deploys it, “does not seem to have violated the law as the Supreme Court has declared it,” Sullivan wrote in the journal for Villanova University's law school. The algorithm “has not engaged in either disparate treatment or disparate impact as those terms have been defined by the court, which has repeatedly described these two theories as if they comprise the entire universe of 'discrimination.'”
Because the artificial intelligence program isn't human, it can't intend to discriminate, and Supreme Court precedent requires intent or motive for what it labels disparate treatment, Sullivan said. In International Brotherhood of Teamsters v. United States, the court said “proof of discriminatory intent is critical,” in an oft-quoted passage.
Disparate impact discrimination might appear a better vehicle to seek redress for unintended discrimination by an algorithm, but there are problems there as well. Disparate impact claims involve employment practices that are facially neutral in their treatment of different groups, but fall more harshly on one group than another, Sullivan wrote. But the catchall doctrine of business necessity serves as a defense to a disparate impact claim. Title VII allows an employer to excuse any disparate impact as job-related, and hire the applicant most likely to perform the job successfully over others less likely to do so, Sullivan said.
A bill introduced in the U.S. Senate and House of Representatives in April would require companies that use “automated decision systems” to facilitate human decision-making that impacts consumers in order to monitor for bias and correct it if found.
Sullivan doubts discrimination law will change anytime soon to accommodate job applicants who suffer artificial intelligence discrimination.
“If we reach the point where society wants to rethink how much it's appropriate to be run by machines, then I think you can expect either Congress or the courts to do something. But I don't expect anything to happen in the near future,” he said.
Sandra Sperino, who teaches employment discrimination law at the University of Cincinnati College of Law, said an overhaul of the courts and legislation may not be needed. Federal anti-discrimination laws and most state laws are written in broad enough terms that they could hold an employer liable for negative outcomes based on a person's protected class.
“The wrinkle is that the courts haven't always stuck to that language. When they've applied the statutes, often they've spoken inartfully in the translation,” Sperino said. “One of the inartful translations is [where] courts will say something like, in order to prove a disparate treatment case the plaintiff must show intent. The problem with that articulation is that intent or motivation is only one way to show that your protected trait caused an outcome.”
A court might rule there's no claim when a machine chooses male applicants over females for reasons we don't understand because the decision can't be linked to human intent. But “that's not what the statute says,” Sperino said. “I think you can show causation there without necessarily showing a particular person's intent.”
Sperino says suits based on failure to hire an applicant are generally challenging for plaintiffs to bring, but she expects the Equal Employment Opportunity Commission to bring suits related to the growth of algorithm-based hiring tools if private litigants struggle with those cases.
In any case involving new technology, “part of the difficult task is always helping the judges to understand what the technology is and whether it's actually different than the cases that don't involve the technology,” Sperino said.
Jason Bent of Stetson University College of Law said anyone suing for algorithmic discrimination will have to recognize they are making a “novel argument.” Bent advocates for a form of “algorithmic affirmative action” to counteract bias in artificial intelligence programs, and believes that use of “race-aware fairness techniques” could survive an equal protection challenge.
Bent notes that the apparent inapplicability of discrimination law to unintentional algorithmic discrimination has prompted a variety of responses. Some have called for modification of the business necessity defense to disparate impact discrimination, while others have proposed a regulatory agency to oversee the use of algorithms.
Developing regulations to govern artificial intelligence “would be a pretty daunting task for that agency,” since algorithms are used in countless ways, Bent said. “If you only focus on employment decision algorithms, that seems more manageable. If you're talking about every use of machine learning in the business context, that's massive. I suppose you could develop a federal agency to regulate that but that would be a pretty expansive task,” he said.
But algorithmic fairness cannot be achieved by hiding protected characteristics from the machine, according to Bent. ”If you give it enough data, the machine will identify some correlations and end up discriminating based on the bias that's reflected in your data,” he said.
“Attempting to build colorblind algorithms is an exercise in futility. Machine learning scholars increasingly agree that the best way to get fair algorithmic results is not by hiding the protected trait, but instead by using the protected trait to set a fairness constraint within the algorithmic design.”
This content has been archived. It is available through our partners, LexisNexis® and Bloomberg Law.
To view this content, please continue to their sites.
Not a Lexis Subscriber?
Subscribe Now
Not a Bloomberg Law Subscriber?
Subscribe Now
NOT FOR REPRINT
© 2024 ALM Global, LLC, All Rights Reserved. Request academic re-use from www.copyright.com. All other uses, submit a request to [email protected]. For more information visit Asset & Logo Licensing.
You Might Like
View All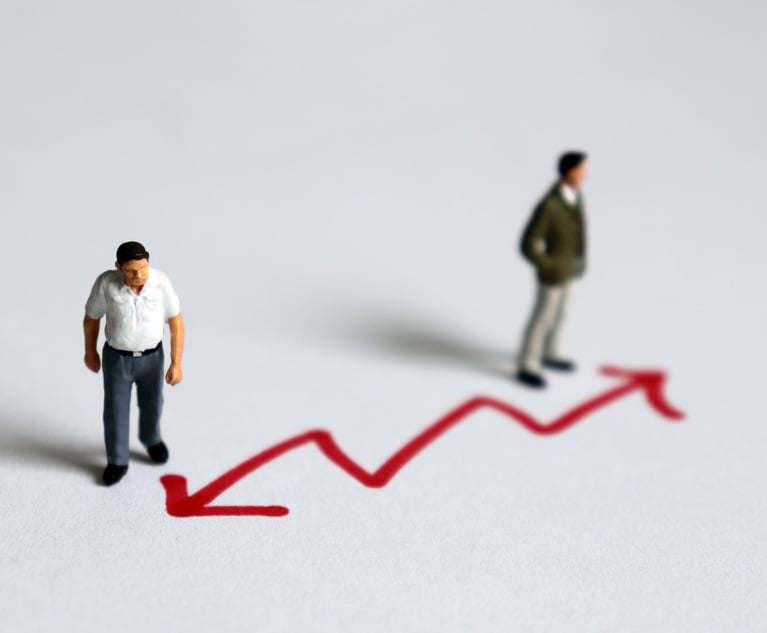

Petitioners Seek to Remove 'Non-Lawyer' From ABA Vocabulary. Lively Discussion Ensues
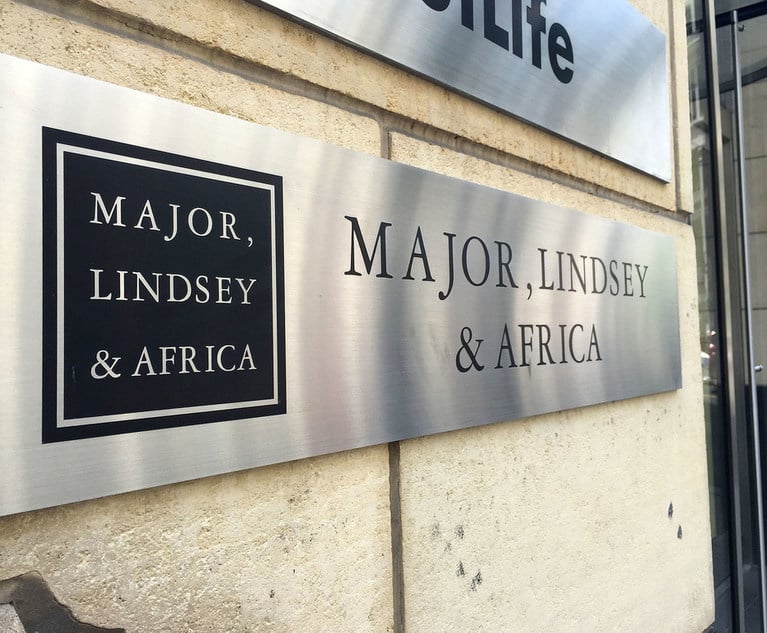
Major Lindsey & Africa 'Blackballed' Associate Who Sued Troutman, Suit Claims

Facing Overcapacity, Law Firms Made Fewer Offers for 2024 Summer Associates
6 minute readTrending Stories
- 1US Magistrate Judge Embry Kidd Confirmed to 11th Circuit
- 2Shaq Signs $11 Million Settlement to Resolve Astrals Investor Claims
- 3McCormick Consolidates Two Tesla Chancery Cases
- 4Amazon, SpaceX Press Constitutional Challenges to NLRB at 5th Circuit
- 5Schools Win Again: Social Media Fails to Strike Public Nuisance Claims
Who Got The Work
Michael G. Bongiorno, Andrew Scott Dulberg and Elizabeth E. Driscoll from Wilmer Cutler Pickering Hale and Dorr have stepped in to represent Symbotic Inc., an A.I.-enabled technology platform that focuses on increasing supply chain efficiency, and other defendants in a pending shareholder derivative lawsuit. The case, filed Oct. 2 in Massachusetts District Court by the Brown Law Firm on behalf of Stephen Austen, accuses certain officers and directors of misleading investors in regard to Symbotic's potential for margin growth by failing to disclose that the company was not equipped to timely deploy its systems or manage expenses through project delays. The case, assigned to U.S. District Judge Nathaniel M. Gorton, is 1:24-cv-12522, Austen v. Cohen et al.
Who Got The Work
Edmund Polubinski and Marie Killmond of Davis Polk & Wardwell have entered appearances for data platform software development company MongoDB and other defendants in a pending shareholder derivative lawsuit. The action, filed Oct. 7 in New York Southern District Court by the Brown Law Firm, accuses the company's directors and/or officers of falsely expressing confidence in the company’s restructuring of its sales incentive plan and downplaying the severity of decreases in its upfront commitments. The case is 1:24-cv-07594, Roy v. Ittycheria et al.
Who Got The Work
Amy O. Bruchs and Kurt F. Ellison of Michael Best & Friedrich have entered appearances for Epic Systems Corp. in a pending employment discrimination lawsuit. The suit was filed Sept. 7 in Wisconsin Western District Court by Levine Eisberner LLC and Siri & Glimstad on behalf of a project manager who claims that he was wrongfully terminated after applying for a religious exemption to the defendant's COVID-19 vaccine mandate. The case, assigned to U.S. Magistrate Judge Anita Marie Boor, is 3:24-cv-00630, Secker, Nathan v. Epic Systems Corporation.
Who Got The Work
David X. Sullivan, Thomas J. Finn and Gregory A. Hall from McCarter & English have entered appearances for Sunrun Installation Services in a pending civil rights lawsuit. The complaint was filed Sept. 4 in Connecticut District Court by attorney Robert M. Berke on behalf of former employee George Edward Steins, who was arrested and charged with employing an unregistered home improvement salesperson. The complaint alleges that had Sunrun informed the Connecticut Department of Consumer Protection that the plaintiff's employment had ended in 2017 and that he no longer held Sunrun's home improvement contractor license, he would not have been hit with charges, which were dismissed in May 2024. The case, assigned to U.S. District Judge Jeffrey A. Meyer, is 3:24-cv-01423, Steins v. Sunrun, Inc. et al.
Who Got The Work
Greenberg Traurig shareholder Joshua L. Raskin has entered an appearance for boohoo.com UK Ltd. in a pending patent infringement lawsuit. The suit, filed Sept. 3 in Texas Eastern District Court by Rozier Hardt McDonough on behalf of Alto Dynamics, asserts five patents related to an online shopping platform. The case, assigned to U.S. District Judge Rodney Gilstrap, is 2:24-cv-00719, Alto Dynamics, LLC v. boohoo.com UK Limited.
Featured Firms
Law Offices of Gary Martin Hays & Associates, P.C.
(470) 294-1674
Law Offices of Mark E. Salomone
(857) 444-6468
Smith & Hassler
(713) 739-1250